引言
空间转录组技术的进展为研究肿瘤微环境中的细胞互作提供了重要见解。该技术产生的数据不仅包含组织的空间基因表达,也涵盖了病理切片信息(H&E image)。目前,许多分析工具都在探索这些数据,主要用于细胞解卷(cell deconvolution)和聚类(clustering)。然而,现有的工具大多只考虑基因表达信息,却忽略了图像中的细胞形态特征。特别是在mRNA捕获率不一的区域,仅依赖基因表达分析难以准确识别不同的区域和细胞类型,从而影响分析的准确性。因此,结合病理切片上的细胞形态学特征与空间基因表达数据,可以更精确地识别肿瘤微环境中的不同区域和细胞类型。
2024年8月25日,埃默里大学(Emory University)的胡健团队与得克萨斯大学MD Anderson肿瘤医院的王凌华团队在Nature Communications上发表论文METI: Deep profiling of tumor ecosystems by integrating cell morphology and spatial transcriptomics,介绍了一种新的空间转录组数据分析框架。胡健和王凌华担任共同通讯作者,博士生蒋嘉汇是该论文的第一作者。
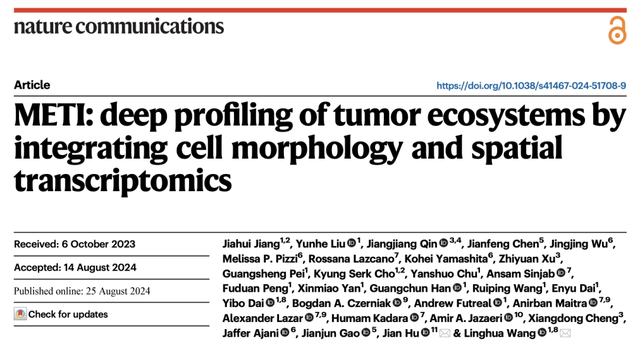
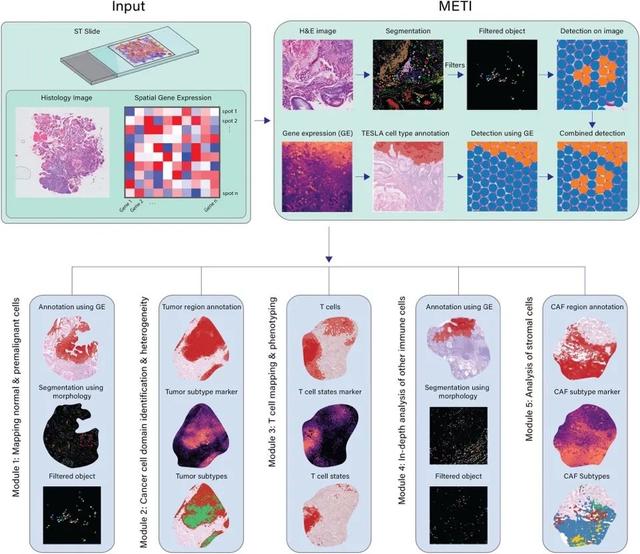
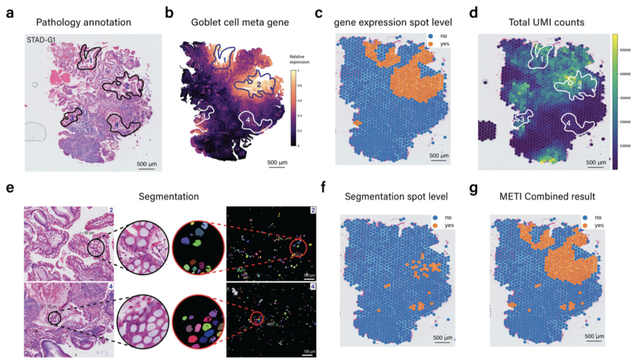
图2:结合图像形态学和基因表达定位杯状细胞(Credit: Nature Communications)
软件链接:https://github.com/Flashiness/METI参考文献
[1] Correa, P., M.B. Piazuelo, and K.T. Wilson, Pathology of gastric intestinal metaplasia: clinical implications. The American journal of gastroenterology, 2010. 105(3): p. 493.[2] Leung, W. and J. Sung, Intestinal metaplasia and gastric carcinogenesis. Alimentary pharmacology & therapeutics, 2002. 16(7): p. 1209-1216.[3] Jencks, D.S., et al., Overview of Current Concepts in Gastric Intestinal Metaplasia and Gastric Cancer. Gastroenterol Hepatol (N Y), 2018. 14(2): p. 92-101.[4] Ohlund, D., et al., Distinct populations of inflammatory fibroblasts and myofibroblasts in pancreatic cancer. J Exp Med, 2017. 214(3): p. 579-596.[5] Elyada, E., et al., Cross-Species Single-Cell Analysis of Pancreatic Ductal Adenocarcinoma Reveals Antigen-Presenting Cancer-Associated Fibroblasts. Cancer Discov, 2019. 9(8): p. 1102-1123.[6] Hu, B., et al., Subpopulations of cancer-associated fibroblasts link the prognosis and metabolic features of pancreatic ductal adenocarcinoma. Ann Transl Med, 2022. 10(5): p. 262.[7] Sahai, E., et al., A framework for advancing our understanding of cancer-associated fibroblasts. Nature Reviews Cancer, 2020. 20(3): p. 174-186.[8] Geng, X., et al., Cancer-Associated Fibroblast (CAF) Heterogeneity and Targeting Therapy of CAFs in Pancreatic Cancer. Front Cell Dev Biol, 2021. 9: p. 655152.[9] Saw, P.E., J. Chen, and E. Song, Targeting CAFs to overcome anticancer therapeutic resistance. Trends Cancer, 2022. 8(7): p. 527-555.[10] Asif, P.J., et al., The role of cancer-associated fibroblasts in cancer invasion and metastasis. Cancers, 2021. 13(18): p. 4720.[11] Feng, B., et al., Cancer-associated fibroblasts and resistance to anticancer therapies: Status, mechanisms, and countermeasures. Cancer Cell International, 2022. 22(1): p. 166.https://www.nature.com/articles/s41467-024-51708-9责编|探索君
排版|探索君
文章来源|“BioArt”
End